are you Ready to dive into the thrilling world of Deep Learning without emptying your wallet? You’re in luck! We’re about to Tell the ultimate roadmap to finding the perfect
affordable GPU for deep learning. budget friendly GPU to kickstart your Deep Learning journey. Let’s break it down into simple easy to understand in depth Guide, shall we?
Why You Need GPUs for Deep Learning
Okay, so Deep Learning is all about crunching tons of data to train your models, right? Well, regular computer brains (CPUs) struggle with this heavy lifting.
That’s where GPUs swoop in! They’re like the superheroes of processing, built to handle these tasks lightning fast. Think quicker training times and less hair pulling frustration for you.
Finding Balancing Cost and Performance
Now, high end GPUs are like Ferraris super speedy but wallet draining. But fear not! For us mere mortals,
it’s all about finding that sweet spot between affordability and power. Here’s what you need to look for:
Memory: Your GPU’s memory (VRAM) is crucial for storing all that juicy data during training. Shoot for at least 6GB, but 8GB or more is even better for larger projects.
Processing Power: More cores and higher clock speeds mean faster calculations. Simple as that!
Software Compatibility: Make sure your GPU plays nice with popular Deep Learning tools like TensorFlow and PyTorch. Most modern GPUs fit the bill.
Top 10 Affordable GPU for Deep Learning
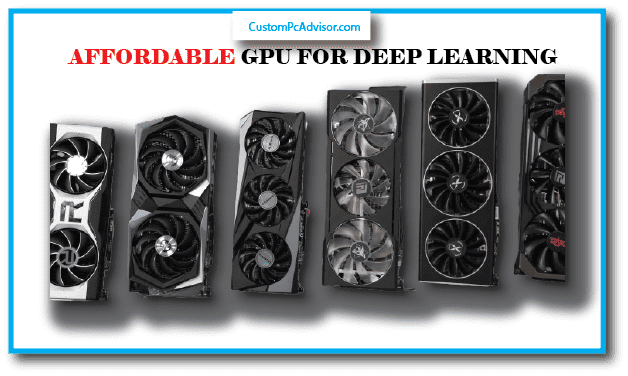
Now, let’s talk specific models that won’t burn a hole in your pocket:
GPU | Price (USD) | Memory (GB) | Strengths for Deep Learning | Weakness |
---|---|---|---|---|
NVIDIA GeForce RTX 3060 Ti | $400-$500 | 8GB | Good balance of performance and price, Tensor Cores for AI workloads | Lower memory compared to higher-end options |
AMD Radeon RX 6700 XT | $350-$450 | 12GB | Competitive price with good memory, strong performance for the cost | May not be optimized for all deep learning frameworks |
NVIDIA GeForce GTX 1660 Super | $250-$300 | 6GB | Budget-friendly option, decent performance for smaller projects | Lower memory and overall processing power |
NVIDIA GeForce RTX 3050 | $200-$300 | 8GB | Entry-level option with some Tensor Core support, good for beginners | Lower performance compared to higher-end RTX cards |
AMD Radeon RX 6600 XT | $250-$350 | 8GB | Budget-friendly AMD option, competes with GTX 1660 Super | May not be optimized for all deep learning frameworks |
NVIDIA GeForce GTX 1080 Ti (Used) | $300-$400 | 11GB | Powerful used option, considerable performance for the price | Higher power consumption, potential warranty limitations |
AMD Radeon RX Vega 64 (Used) | $200-$300 | 16GB | Large memory capacity for the price (used), good for specific workloads | Older architecture, higher power consumption |
NVIDIA Titan Xp (Used) | $400-$500 | 12GB | Very powerful used option, excellent performance for the price | Very high power consumption, potential warranty limitations |
Intel Arc A770 | $350-$400 | 16GB | New entry from Intel, promising performance at a good price | Limited testing data for deep learning workloads yet |
NVIDIA GeForce GTX 1650 | $150-$200 | 4GB | Most affordable option, suitable for basic deep learning tasks | Very low memory, limited for complex projects |
More Important Harwares Needs for Deep Learnings
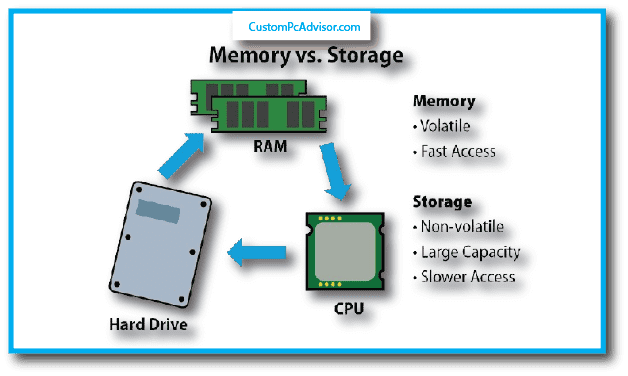
Don’t forget, your GPU isn’t the only player in this game:
CPU: Get yourself a decent CPU with at least 4 cores and snappy clock speeds.
RAM: Aim for 16GB or more to keep things running smoothly.
Storage: SSDs are your best friends here, speeding up data access and training times.
Deep Learning Starting Strong on a Budget
Ready to kick off your Deep Learning journey? Here’s how to hit the ground running:
Start Small: Don’t bite off more than you can chew. Begin with simpler projects that match your GPU’s capabilities.
Embrace the Cloud: Many platforms offer free GPU instances perfect for experimenting without spending a dime upfront.
Learn, Learn, Learn: Master the basics of Deep Learning to make the most of your hardware, no matter its limitations.
What factors to consider besides price?
Apart from looking at the price, think about how much memory the GPU has (measured in GB), how fast it can do calculations, and if it works well with the software you’re planning to use, like TensorFlow or PyTorch.
What are Tensor Cores?
Tensor Cores are special parts inside NVIDIA GPUs that make certain calculations used in deep learning faster, which makes the whole process work better.
Is higher memory (GB) always better?
Having more memory (GB) in a GPU is usually good, especially if you’re dealing with big amounts of data or complicated stuff. But if your project is simple, you might not need as much memory.
Advantages and disadvantages of used GPUs?
Buying a used GPU can save you a lot of money, which is great. But sometimes, used ones might not have a warranty or they could use up more power.
AMD vs NVIDIA for deep learning?
Choosing between AMD and NVIDIA GPUs depends. NVIDIA GPUs have Tensor Cores that help with deep learning, and they’re usually well matched with popular software. AMD GPUs
can be strong performers and cheaper, but it’s a bit of a toss up. It depends on what you need and what you’re comfortable with.
RTX 3060 Ti vs. RX 6700 XT?
When comparing the RTX 3060 Ti and the RX 6700 XT, both are strong choices. The RTX 3060 Ti tends to perform slightly better, thanks to its Tensor Cores, which help with deep
learning tasks. However, the RX 6700 XT often comes with more memory at a similar price point. To make the best decision, look into benchmarks for the specific tasks you’ll be doing.
GTX 1660 Super for small image recognition?
Absolutely, the GTX 1660 Super can be a solid choice for starting out with smaller image recognition projects. It strikes a good balance between price and performance, making it accessible for beginners.
RTX 3050 for beginners?
The RTX 3050 is a great entry level option, particularly for beginners diving into deep learning. With some Tensor Core support,
it offers a decent starting point for learning the basics and tackling less complex projects effectively.
Memory vs Newer Model (on a tight budget)?
If you’re on a tight budget, deciding between more memory and a newer model depends on your specific needs. For smaller datasets or less demanding projects, opting for a newer
model with less memory could be sufficient. However, if you’re dealing with larger datasets, prioritize more memory, even if it means considering an older card.
Used GTX 1080 Ti vs. RX Vega 64?
Both the used GTX 1080 Ti and the RX Vega 64 are powerful options. The GTX 1080 Ti typically offers better performance, while the RX Vega 64 often boasts more memory.
To make an informed decision, research benchmarks tailored to your workload to determine which card aligns better with your requirements.
Considering future software updates?
Newer GPUs may receive better support from future deep learning software updates, but don’t count out older models entirely.
Some older GPUs still perform admirably and might meet your needs.
Researching GPU performance with a framework?
To gauge GPU performance accurately, look for online benchmarks tailored to the specific deep learning framework you intend to use.
These benchmarks provide real world performance insights that can guide your decision.
New and upcoming affordable GPUs?
Keep an eye on the Intel Arc series for affordable GPUs offering promising performance. Checking reviews, especially concerning their suitability for deep learning applications,
can provide valuable insights.
Power consumption of deep learning GPUs?
Deep learning GPUs can consume a significant amount of power, so consider electricity costs when selecting a GPU.
Ensure your power supply can handle the GPU’s power demands to avoid any issues.
Other hardware for deep learning tasks?
In addition to GPUs, a robust CPU with ample RAM is crucial for deep learning tasks. CPUs handle various computations alongside the GPU, contributing to overall performance and
efficiency. Make sure your system is well balanced to optimize deep learning workflows effectively.
Absolute cheapest option for basic deep learning?
If you’re on a tight budget, the GTX 1650 is among the most affordable options capable of handling basic deep learning tasks.
Cloud computing for deep learning?
Cloud services provide access to robust GPU resources for deep learning projects, making them a viable alternative if you prefer not to invest in physical GPUs upfront.
Can CPUs handle deep learning?
While CPUs can manage basic deep learning tasks, they’re significantly slower than dedicated GPUs, particularly for complex projects. For optimal performance, GPUs are generally preferred.
Financing options for GPUs?
Some retailers offer financing plans for purchasing GPUs, allowing you to spread out the cost over time.
Be sure to research and compare terms from different providers before making a commitment.
Conclusion
Choosing the right GPU for deep learning on a budget can be tough. This table can help you get started and understand your options. Find a balance between memory (GB) and price
that works for you. Don’t assume newer is always better some used GPUs can give you good performance for less money. Make sure to research how each GPU handles the type
of deep learning you’re interested in. And keep an eye out for deals because prices can change!
FAQs
How much VRAM do I really need?
The VRAM you need depends on how complex your deep learning projects are. If you’re new or working with small datasets, 6GB of VRAM is a good start. But if you’re dealing with larger models or datasets, aim for 8GB or more.
Are Nvidia and AMD GPUs different for deep learning?
Both Nvidia and AMD have GPUs for deep learning. Nvidia is popular because its GPUs are optimized for deep learning frameworks like TensorFlow and PyTorch. AMD GPUs can be good too, especially if they offer good performance for the price.
Is it better to buy new or used GPUs for deep learning?
It depends on your budget and how much risk you’re willing to take. New GPUs are more expensive but offer the latest features and performance. Used GPUs can be cheaper but might have issues like slower performance over time. If you buy used, make sure the seller is reputable and offers a warranty.
Can I use my laptop for deep learning?
Some high end gaming laptops have powerful GPUs that can work for deep learning. But they can be pricey and might not handle long training sessions well due to heat.
Where can I learn deep learning for free?
There are lots of free resources online! You can try platforms like Coursera, edX, and Udacity for courses, check out tutorials and documentation from TensorFlow and PyTorch, or find deep learning tutorials on YouTube.
Any tips for saving money on a deep learning setup?
Sure! You can build your own PC to save money and have more control over components. Keep an eye out for deals and discounts on GPUs and other hardware. And don’t feel like you need to buy the most expensive stuff right away start with something affordable and upgrade later.